Annotation Tools for Machine Learning: Unlocking New Possibilities
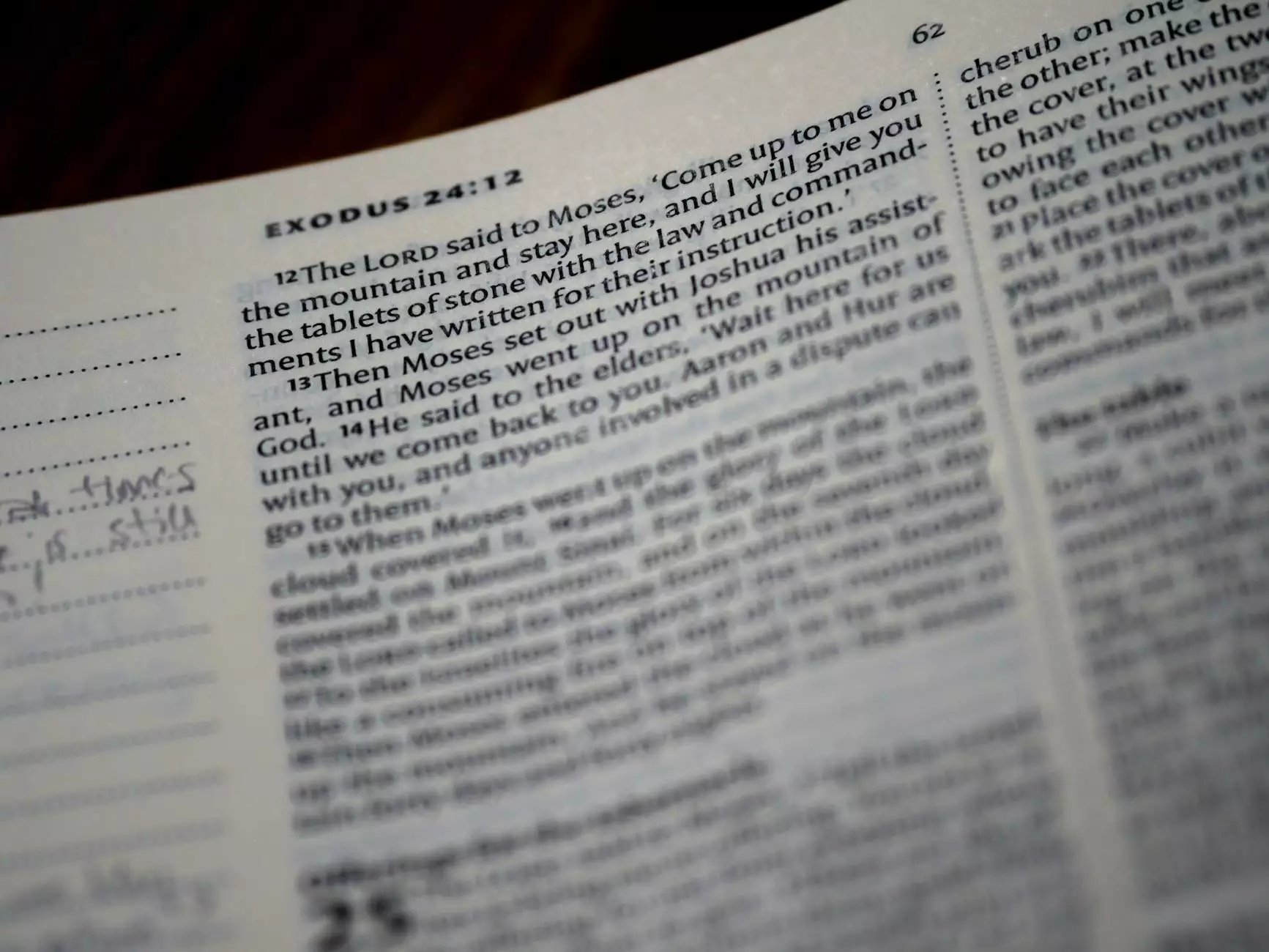
In the dynamic world of machine learning, data is the cornerstone of innovation. To leverage data effectively, businesses must invest in high-quality labeling and annotation. Annotation tools for machine learning provide the necessary framework to transform raw data into valuable insights. In this article, we will explore the significance of these tools, the different types available, and how they can enhance your business operations, particularly in the realms of Home Services and Keys & Locksmiths.
The Importance of Data Annotation in Machine Learning
Data annotation involves the process of labeling data to train machine learning models. This process is crucial because algorithms learn from labeled data to make predictions and classifications. Without accurate annotations, the reliability of the models can be compromised, leading to poor performance in real-time applications.
- Enhanced Model Accuracy: Properly annotated data allows models to learn effectively, resulting in improved accuracy.
- Time Efficiency: Automated annotation tools can significantly reduce the time it takes to annotate large datasets.
- Scalability: As businesses grow, so does the amount of data they accumulate. Quality annotation tools can scale according to business needs.
Types of Annotation Tools for Machine Learning
Different types of annotation tools for machine learning cater to various data types and project requirements. Here are some of the most popular annotation tools:
1. Image Annotation Tools
Image annotation tools are essential for projects involving computer vision. These tools help label images for object detection, classification, and segmentation tasks. Common functionalities include:
- Bounding boxes for object detection.
- Polygons for precise segmentation.
- Landmark annotation for facial recognition.
2. Text Annotation Tools
In natural language processing (NLP), text annotation tools are instrumental for sentiment analysis, entity recognition, and more. Common features include:
- Named Entity Recognition (NER) for identifying entities within text.
- Sentiment tagging to assess the emotional tone of the text.
- Part-of-Speech (POS) tagging for linguistic purposes.
3. Audio Annotation Tools
Audio annotation tools are tailored for machine learning projects that involve speech recognition or sound analysis. Functionalities often include:
- Transcription of spoken words into text.
- Labeling sounds for classification tasks.
4. Video Annotation Tools
For projects that require video analysis, video annotation tools play a crucial role. Features might include:
- Object tracking across video frames.
- Event tagging for specific actions occurring in the video.
Benefits of Using Annotation Tools for Machine Learning
Implementing annotation tools for machine learning brings numerous advantages to businesses looking to harness the power of artificial intelligence:
1. Improved Data Quality
High-quality data is paramount for reliable machine learning outcomes. Automated annotation tools minimize human error and maintain consistency across annotations, ensuring your model trains on the best possible dataset.
2. Cost-Effective Solutions
By automating parts of the annotation process, businesses can save significant time and resources. This optimization allows teams to focus on other critical tasks, ultimately enhancing productivity and reducing operational costs.
3. Robust Collaboration Features
Many annotation tools come equipped with features that facilitate collaboration among team members. These tools allow for real-time feedback, project management, and version control, fostering a more efficient workflow.
4. Versatility Across Different Domains
Annotation tools are versatile and can be tailored for various domains, including healthcare, automotive, agriculture, and more. This adaptability makes them ideal for businesses operating in diverse sectors.
Choosing the Right Annotation Tool for Your Business
Selecting the right annotation tool for machine learning can be daunting. Here are some essential factors to consider:
- Data Type: Ensure the tool supports the specific data types you are working with, whether images, text, audio, or video.
- User Interface: A user-friendly interface increases adoption rates and reduces the learning curve for teams.
- Integration Capability: Check if the tool can integrate seamlessly with your existing systems and workflows.
- Customization: The ability to customize annotation settings and workflows can be a significant advantage tailored to your business needs.
Implementing Annotation Tools in the Home Services Sector
In the Home Services industry, machine learning applications can significantly enhance operational efficiency. Here are a few examples of how annotation tools can be leveraged:
1. Customer Support Analysis
By annotating customer support interactions, businesses can train models to identify and categorize customer issues. This process can lead to improved response times and enhanced customer satisfaction.
2. Predictive Maintenance
Annotating data from equipment sensors and service history can help develop predictive maintenance models. These models can forecast potential failures, reducing downtime, and service costs.
Annotation Tools for Locksmiths: Enhancing Security and Customer Experience
In the Keys & Locksmiths industry, machine learning can revolutionize service delivery. Here’s how annotation tools can play a role:
1. Smart Lock Technology
As smart lock technology advances, machine learning models trained with accurately annotated data can better identify unauthorized access attempts and enhance security measures.
2. Customer Behavior Analysis
Annotating customer interaction data can assist locksmith businesses in understanding consumer preferences and patterns, allowing for tailored service offerings and marketing strategies.
Future Trends in Data Annotation for Machine Learning
As technology advances, so too does the field of data annotation. Here are some emerging trends to watch for in the future:
1. Increased Automation
With the advancement of AI and machine learning technologies, we will likely see more automated annotation tools that can handle vast amounts of data efficiently and accurately.
2. Crowdsourcing Approaches
Crowdsourcing for data annotation is gaining traction, enabling businesses to tap into a broader pool of annotators, thereby increasing speed and reducing costs.
3. Enhanced Collaboration Tools
Future annotation tools will likely come with more sophisticated collaboration features, allowing for seamless workflow management and communication among team members regardless of location.
Conclusion
In conclusion, annotation tools for machine learning are vital instruments that can transform raw data into actionable insights, driving innovation and efficiency within businesses. For industries like Home Services and Keys & Locksmiths, the potential applications are vast, paving the way for enhanced customer experiences, improved service delivery, and informed decision-making.
As businesses continue to evolve in a data-driven landscape, investing in quality annotation tools will undoubtedly be a critical factor in maintaining competitiveness and fostering growth. Embrace these tools, as they will unlock new possibilities for your business, enabling you to rise above the competition and achieve long-term success.